3. Solve the following linear programming model graphically: Maximize \( \mathrm{Z}=3 \mathrm{X}_{1}+6 \mathrm{X}_{2} \) Subject to: \( 3 \mathrm{X}_{1}+2 \mathrm{X}_{2} \leq 18 \) \( \mathrm{X}_{1}+\mathrm{X}_{2} \geq 5 \) \( \mathrm{X}_{1} \leq 4 \) \( \mathrm{X}_{1}, \mathrm{X}^{2} \geq 0 \)
Solución de tutoría real
Responder
Solución

¡Inicia sesión para desbloquear respuestas gratis!
Una plataforma de aprendizaje en la que confían millones de estudiantes y profesores reales.

Revisado y aprobado por el equipo de tutoría de UpStudy
Extra Insights
To tackle this linear programming problem graphically, start by plotting the constraints on a coordinate plane with \( \mathrm{X}_{1} \) on the x-axis and \( \mathrm{X}_{2} \) on the y-axis. 1. **Constraints**: - From \( 3 \mathrm{X}_{1} + 2 \mathrm{X}_{2} \leq 18 \), rearrange to find the line: \( \mathrm{X}_{2} = 9 - 1.5 \mathrm{X}_{1} \). - From \( \mathrm{X}_{1} + \mathrm{X}_{2} \geq 5 \), rearrange to find the line: \( \mathrm{X}_{2} = 5 - \mathrm{X}_{1} \). - The line \( \mathrm{X}_{1} = 4 \) is vertical. - \( \mathrm{X}_{1}, \mathrm{X}_{2} \geq 0 \) keeps you in the first quadrant. 2. **Feasible Region**: Shade the area that satisfies all constraints (below the first line and above the second line, while respecting \( \mathrm{X}_{1} \leq 4 \) restriction). 3. **Objective Function**: Plot the lines of the objective function \( \mathrm{Z} = 3 \mathrm{X}_{1} + 6 \mathrm{X}_{2} \) for various values of \( Z \). The value of \( Z \) increases as you move parallel to this line. 4. **Finding Maximum**: Identify the vertices of the feasible region (corners) and evaluate the objective function \( \mathrm{Z} \) at each vertex to find which one gives the maximum value. Continuing from here will lead you to the optimal solution! Now let’s add some interesting context to this topic! The world of linear programming has roots back in the 1940s during World War II, primarily used for resource allocation problems in military logistics. The simplex method, developed by George Dantzig, enabled the efficient optimization of complex problems, making it essential in various sectors, including economics, engineering, and transportation. In real life, linear programming isn't just academic; it's actively used across industries! Companies apply these techniques for everything from optimizing production processes to minimizing costs in supply chain management. Picture a delivery company using linear programming to determine the most efficient routes to ensure timely deliveries while saving on fuel—now that’s a practical application making a difference!
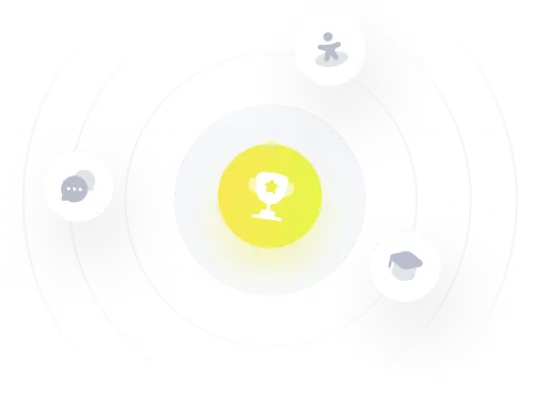